NLP
Executive Summary
The NLP section focuses on analyzing the trends in submission posts and comments of the nine subreddits gathered, seven related to politics and two related to economics. In these nine subreddits, we uncovered key insights about the overall text distributions and sentiments expressed by its users in both submissions and comments. The main focus, however, is on sentiment analysis, which was conducted using a pre-trained sentiment model from the johnsnowlabs sparkNLP
library. With its help, we assigned an overall sentiment of positive, negative, or neutral to each submission and comment.
The results of our analysis revealed that there is a decent amount of overlap in the top 100 words present in the politics and economics subreddits comments. We also found major dips in activity in economics subreddits comments, which could be attributed to U.S or global major events in December 2021. In terms of sentiment, we found that r/Conservative
, r/Libertarian
, and r/centrist
contain higher positive sentiment in comments, while r/Economics
and r/Liberal
contain higher negative sentiment. In submissions, r/AskPolitics
and r/Conservative
contain higher negative sentiment, while r/Economics
and r/Liberal
contain higher positive sentiment. We also found that the majority of comments and submissions are positive, with a significant amount of negative sentiment. This suggests that while there is a prevailing positive discourse, a substantial amount of the conversation is polarized, with negative comments forming a notable part of the dialogue. The low percentage of neutral comments could indicate that participants in these discussions tend to take a clear stance rather than a neutral one.
We also conducted sentiment analysis on the r/Economics
subreddit using external data from the fred
python package The results revealed a significant spike in the frequency of “recession” mentions in the r/Economics
subreddit, which aligns closely with key economic events. This suggests that the subreddit’s users are highly responsive to real-world economic indicators and news, and underscores the role of major economic announcements in shaping public discourse.
Finally, LDA Topic Modeling was conducted separately on the submissions of the seven political subreddits and two economics subreddits and it illuminated distinct themes within political and economics subreddits. In politics, discussions ranged from exploring beliefs and perspectives, dissecting political parties and national contexts, to examining government policies and societal initiatives. These encompassed global affairs, societal issues, and ideological perspectives on political systems. In economics, topics spanned global economic and political interconnectedness, discussions on oil protests, housing markets, cryptocurrencies, Biden administration’s efforts in student loan accessibility, trade dynamics, and the fusion of technology and finance through fintech applications.
Analysis Report
Business Goal #8: Basic NLP and Text Checks.
We created an NLP Pipeline built with johnsnowlabs
and the spark-nlp
package. The following functions from the package were utilized on both submissions, including the selftext
and title
columns, and comments, including the body
column, datasets:
DocumentAssembler()
: Transforms input data into Spark NLP annotated documents.Tokenizer()
: Splits the text into individual words, also known as tokens.Normalizer()
: Performs various text normalization techniques, such as converting text to lowercase, removing special characters, and replacing abbreviations with their full forms.NorvigSweetingModel.pretrained()
: Applies a spell checker based on the Norvig and Sweeting algorithm to correct spelling mistakes.StopWordsCleaner()
: Removes common stop words from the text, such as “the”, “and”, “a”, etc., which are generally not helpful in determining the sentiment of a text.LemmatizerModel.pretrained()
: Reduces each word to its base form (lemma), which can improve the consistency of analysis by grouping together related words.Finisher()
: Extracts the processed text from the Spark NLP document and formats it as a regular string for further analysis.
Frequent Words
Rank | Word | Word Count |
---|---|---|
1 | people | 71,173 |
2 | cmv (Change My View) | 60,735 |
3 | Biden | 50,499 |
… | … | … |
9 | Trump | 33,879 |
… | … | … |
13 | right | 27,886 |
14 | state | 27,344 |
… | … | … |
29 | government | 19,999 |
… | … | … |
42 | democrat | 16,373 |
43 | country | 15,758 |
44 | man | 15,494 |
45 | republic | 15,333 |
… | … | … |
53 | conservative | 13,856 |
54 | find | 13,637 |
55 | law | 13,603 |
56 | party | 13,541 |
… | … | … |
59 | police | 13,263 |
60 | mean | 13,216 |
61 | election | 13,151 |
… | … | … |
73 | America | 12,469 |
74 | libertarian | 12,465 |
… | … | … |
99 | gun | 10,698 |
100 | tax | 10,677 |
Table 1 above shows the top 100 most frequent words in the submissions of the seven political subreddits. We can see that users predominantly discuss a variety of political topics. The top ten most frequently used words suggest a focus on individuals (“people”), the Change My View (CMV) subreddit, and prominent political figures such as Joe Biden and Donald Trump. Other notable themes include discussions about political ideologies (“right,” “conservative,” “libertarian”), governmental entities (“government,” “state”), and political processes (“election”). Additionally, terms like “democrat,” “republic,” and “country” indicate discussions related to political parties and the broader national context. Finally, the presence of words like “police,” “gun,” and “tax” suggests discussions on law enforcement, firearms, and taxation policies. Overall, the word frequency analysis indicates a diverse range of political topics and perspectives within these subreddits, reflecting the complexity and breadth of political discourse on political subreddits.
Note: Tables for other subreddits are in the appendix.
Top 10 Important Words Quantified by TF-IDF
Rank | Word | TF-IDF Value |
---|---|---|
1 | baathism | 99.91752355387538 |
2 | sunscreen | 99.91752355387538 |
3 | stormcloaks | 99.9059313812928 |
4 | subjectivism | 99.9059313812928 |
5 | breakdancing | 99.9059313812928 |
6 | thalmor | 99.9059313812928 |
7 | mdici | 99.9059313812928 |
8 | neopaganism | 99.9059313812928 |
9 | haganah | 99.9059313812928 |
10 | akilensai | 99.9059313812928 |
Note: Tables for other subreddits are in the appendix.
Distribution of Text Length
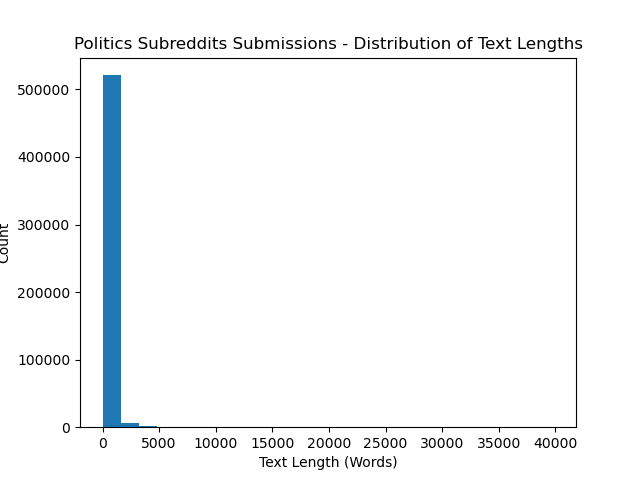
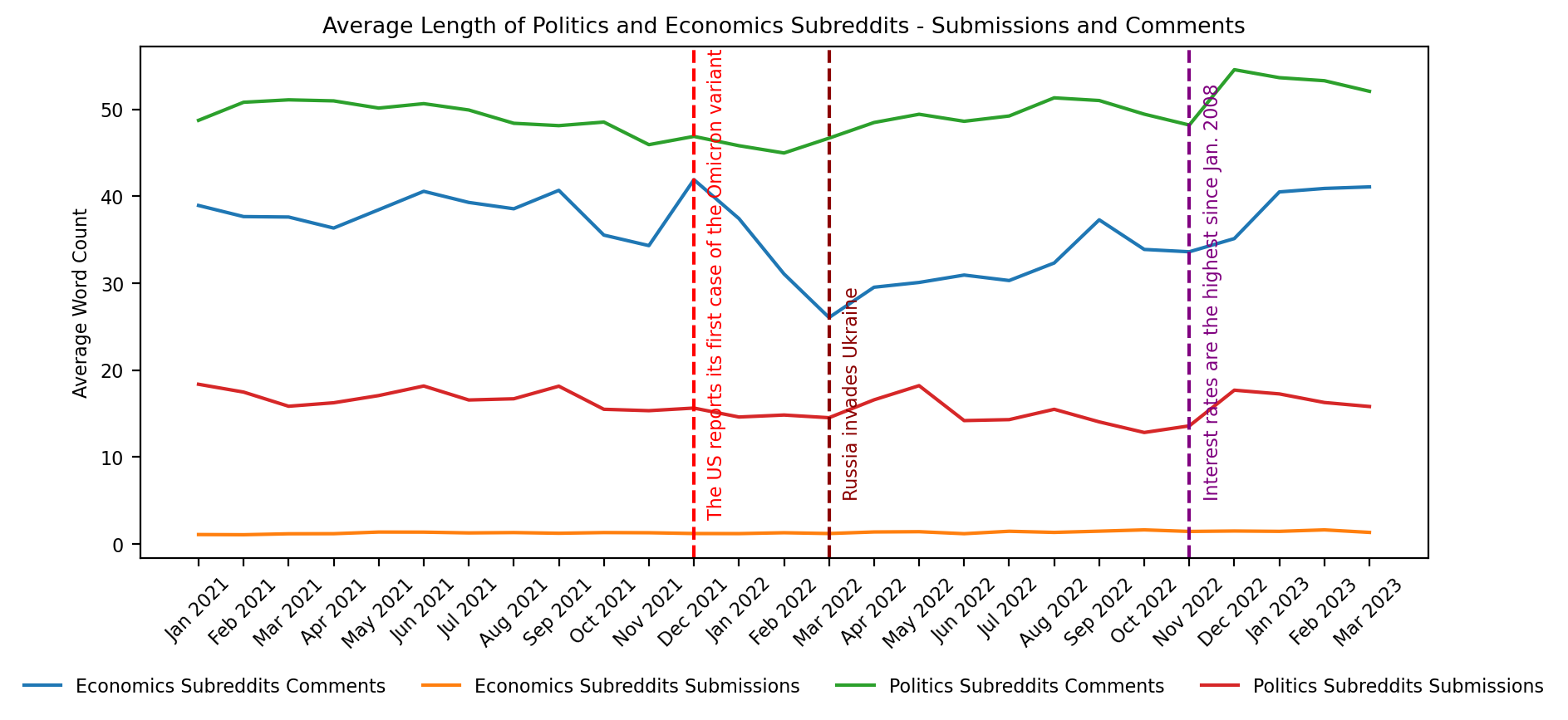
Figure 1 (and all histograms similar to it in the appendix) denote large outliers for submissions and comments in the subreddits (with Figure 1 focusing on Politics subreddits), and a majority of submissions and posts have text lengths between 0 to 500 words. In figure 2, however, we see a massive drop in average word count from 40 to almost 25 in the economics subreddits’ comments from December 2021 to March 2022. A number of major events took place in the U.S around those dates and could have been the cause of the drop in average word count. The events include the Supreme Court hearing arguments on the Texas abortion law and COVID-19 updates on the Omicron virus. Activity for political subreddits’ submissions and comments seem to be relatively stable, with a slight peak in submissions in May 2022.
Business Goal #9: Assess the Mood and Attitude in Posts and Comments across Subreddits in Relation to the U.S. GDP.
Table 3 presents sentiment analysis data for various political subreddits. Notably, ‘Conservative’ and ‘Libertarian’ subreddits exhibit a higher proportion of positive comments, with 57.44% and 58.44%, respectively. In contrast, the ‘Economics’ subreddit shows a balance between positive and negative sentiments, with negative comments slightly edging out at 57.22%. The ‘Liberal’ subreddit has a relatively even distribution of positive and negative sentiments, but negative comments are more prevalent at 52.59%. ‘Centrist’ stands out with the highest percentage of positive comments at 60.16%. Each subreddit demonstrates a unique sentiment profile, which could reflect the general attitudes and discussions prevalent in these online communities.
Table 4 summarizes the overall sentiment of comments, showing that the majority (57.85%) are positive, while a significant portion (38.14%) are negative. Neutral comments constitute the smallest group at 4.02%. This distribution suggests that while there is a prevailing positive discourse, a substantial amount of the conversation is polarized, with negative comments forming a notable part of the dialogue. The low percentage of neutral comments could indicate that participants in these discussions tend to take a clear stance rather than a neutral one.
Table 5 provides data on the sentiment of submissions across various political subreddits. In r/AskPolitics
, the distribution is almost evenly split between positive and negative submissions, with negligible neutral content. r/Conservative
submissions lean more towards the positive at 51.23%, but also have a substantial proportion of negative sentiment at 44.87%. The r/Economics
subreddit is predominantly positive at 56.97%, with negative submissions also forming a significant part at 40.05%. ‘Liberal’ submissions are mostly positive at 54.37%. Overall, while positive submissions prevail across the subreddits, there is a notable presence of negative sentiment, reflecting the contentious nature of political discourse on these platforms.
Table 6 shows the sentiment distribution of total submissions, where positive sentiments constitute the majority with 54.28%. Negative sentiments are also prominent, comprising 42.02% of submissions, which points to a significant level of divisiveness or critical discourse. Neutral sentiments are relatively rare, making up only 3.71% of the total, suggesting that most contributors have definitive opinions that are either positive or negative, rather than neutral. This distribution highlights a polarized environment where neutral or ambivalent voices are far less common compared to those expressing clear-cut positive or negative stances.
r/centrist
Submissions
Figure 3 above (and the similar ones in the appendix) showcases the relationship between U.S. Real GDP and the number of posts categorized by sentiment (positive, neutral, and negative) from various political subreddits over time. The line graph represents the trend in Real GDP, which seems to rise steadily in most charts before plateauing or contracting, indicating a period of economic downturn. Bar graphs display the sentiment of posts in the respective subreddits, with the volume of posts often showing fluctuations.
From a cross-comparison among the subreddits, it seems that the trends in sentiment and GDP do not correspond uniformly across different political spectra. The sentiment analysis from these subreddits could potentially reflect the general mood or economic outlook of their members, with varying degrees of positive, neutral, and negative posts. Notably, there are points where an increase in negative sentiment coincides with a contraction in GDP, which could suggest a correlation between economic performance and public sentiment as expressed in these online communities. However, without a deeper statistical analysis, it cannot be conclusively stated that there is a causal relationship.
r/Economics
subreddit with mentions of “recession” vs U.S Real GDP
As discussed in the EDA section, we gathered external data from the fred python package. The bar chart above depicts the frequency of “recession” mentions in the r/Economics
subreddit reveals a significant pattern that aligns closely with key economic events. Initially, the mentions of “recession” were extremely low, indicating a period of less concern or focus on economic downturns among the subreddit’s discussions. This phase reflects a period of either economic stability or a lack of immediate economic threats that would trigger widespread discussions about a recession.
However, a notable change occurs around the third quarter of 2022. During these months, the mentions of “recession” spiked dramatically. This increase in discussions around recession correlates strongly with a significant real-world economic event: the United States announcing that its Gross Domestic Product (GDP) contracted for two consecutive quarters. This is a classic technical indicator of a recession, which understandably would spark increased interest and concern in an economics-focused community.
The timing of this spike in conversation suggests that the subreddit’s users are highly responsive to real-world economic indicators and news. It also underscores the role of major economic announcements in shaping public discourse. The heightened focus on the term “recession” during these months likely reflects a mix of concern, analysis, and speculation about the future of the economy, both in the U.S. and globally.
Business Goal #10: Determine Prevalent Subjects or Themes in Combined Political and Economics Subreddits Submissions.
The Latent Dirichlet Allocation (LDA)
Model is a statistical method for uncovering hidden (latent) topics within a set of documents. It assumes that each document consists of a blend of a limited number of topics, and each topic is essentially a probability distribution across words. The model functions by portraying each document as a probability distribution over topics and each topic as a probability distribution over words. Through the examination of these distributions, LDA can pinpoint the most significant topics related to the documents in a collection, even if these topics are not explicitly expressed in the documents.
In this section, we aim to determine the prevalent subjects or themes of the combined seven political subreddits and two economics subreddits submissions. We first employed the LDA
model from the pyspark.ml.clustering
class in Azure ML and then the LdaModel
from gensim.models.ldamodel
to conduct topic modeling on the submissions and comments of the nine subreddits. The gensim
library was used to generate the interactive visualization, created with the pyLDAvis
package, below for our topics as pyspark
does not have a visualization tool for LDA models. However, both pyspark
and gensim
outputted similar topics and the topics generated with gensim
are presented below.
Also note that when creating the LDA model, we set the number of topics to 5.
Political Subreddits Submissions
The table below summarizes Figure 5 shown above:
Topic | Topic Words | Summary |
---|---|---|
1 | people, think, want, good, right, cmv (change my view), life, use, change, believe | Exploring perspectives and beliefs in political discourse to understand and potentially alter their own opinions (similar to Table 3 above) |
2 | widen (Biden), Trump, cmv (change my view), democrat, republican, conservative, vote, gun, party, bill | Discussions related to political parties and the broader national context (similar to Table 1 above) |
3 | state, government, work, school, free, child, money, social, public, give, allow | Discussion on government policies and societal initiatives with a focus on financial support and accessibility |
4 | covid, white, house, police, election, vaccine, abortion, death, law, crime, russia, military, justice | Discussions encompassing mournful local and global affairs as well as societal issues |
5 | libertarian, socialist, leftist, war, power, politics, history, story, ukraine, afghanistan, russian | Ideological perspectives on political systems with with a focus on historical narratives and geopolitical events in war-torn regions |
Economics Subreddits Submissions
The table below summarizes Figure 6 shown above:
Topic | Topic Words | Summary |
---|---|---|
1 | stock, inflation, market, rate, change, economy, high, world, china, covid, ukraine, russia | Discussions highlighting the interconnectedness of global economic and political events with financial markets |
2 | price, crypto, rise, tax, house, work, million, bitcoin, investor, job, uk, oil, gas | Discussions related to oil protests in the UK, housing markets, and cryptocurrencies |
3 | new, year, buy, people, loan, credit, help, widen (Biden), claim, report, student | Discussion on Biden administration’s efforts to expand student loan access and financial assistance for borrowers |
4 | make, money, trade, business, currency, weakness, strength, payment | Discussions on trade and currency dynamics influencing global economic strength and business opportunities |
5 | fintechinshorts, fintechnews, finance, fund, debt, investment, bill, partner, risk, asset, app | Discussing the convergence of technology and finance, while underscoring the collaborative and user-centric approach of fintech as well as risk management |
Appendix
Rank | Word | Word Count |
---|---|---|
1 | fintechinshorts | 7,003 |
… | … | … |
5 | bank | 3,810 |
… | … | … |
10 | market | 2,885 |
11 | inflation | 2,784 |
12 | stock | 2,537 |
… | … | … |
17 | rate | 16,373 |
18 | money | 2,121 |
19 | man | 2,116 |
20 | get | 2,074 |
21 | price | 1,958 |
22 | good | 1,943 |
23 | economy | 1,937 |
24 | review | 1,850 |
25 | finance | 1,790 |
… | … | … |
30 | economic | 1,448 |
31 | crypto | 1,429 |
32 | trade | 1,429 |
33 | world | 1,429 |
… | … | … |
38 | covid | 1,310 |
39 | buy | 1,301 |
40 | global | 1,298 |
41 | weakness | 1,296 |
… | … | … |
51 | rise | 1,234 |
52 | help | 1,225 |
53 | pay | 1,225 |
54 | work | 1,203 |
55 | time | 1,200 |
56 | fund | 1,124 |
… | … | … |
96 | account | 854 |
97 | growth | 854 |
98 | fall | 851 |
99 | crisis | 846 |
100 | recession | 833 |
Rank | Word | Word Count |
---|---|---|
1 | people | 3,916,872 |
2 | like | 2,429,385 |
3 | say | 2,388,813 |
4 | get | 2,383,236 |
5 | think | 2,212,302 |
… | … | … |
28 | state | 938,986 |
29 | we | 927,029 |
30 | year | 828,483 |
31 | government | 828,131 |
… | … | … |
48 | Trump | 655,714 |
49 | actually | 652,720 |
50 | look | 650,575 |
51 | vote | 644,104 |
52 | country | 627,094 |
… | … | … |
59 | pay | 583,674 |
60 | post | 573,795 |
61 | law | 572,163 |
62 | reason | 565,496 |
… | … | … |
77 | child | 506,417 |
78 | money | 504,222 |
79 | argument | 504,091 |
80 | live | 500,994 |
… | … | … |
98 | yes | 433,362 |
99 | wrong | 428,341 |
100 | keep | 427,201 |
Rank | Word | Word Count |
---|---|---|
1 | people | 274,244 |
2 | make | 215,993 |
3 | get | 209,560 |
4 | go | 194,391 |
… | … | … |
7 | pay | 143,362 |
8 | money | 141,992 |
9 | work | 141,738 |
10 | think | 138,345 |
11 | say | 134,141 |
12 | price | 128,054 |
13 | tax | 127,177 |
… | … | … |
19 | inflation | 110,184 |
20 | rate | 109,396 |
21 | time | 106,155 |
22 | market | 103,920 |
23 | even | 103,606 |
24 | house | 103,563 |
25 | high | 99,101 |
… | … | … |
37 | job | 81,568 |
38 | buy | 80,449 |
39 | company | 79,214 |
40 | way | 78,705 |
41 | increase | 78,237 |
42 | government | 78,082 |
… | … | … |
52 | country | 64,732 |
53 | interest | 64,663 |
54 | lot | 64,473 |
55 | home | 64,098 |
56 | economy | 64,068 |
57 | right | 63,889 |
58 | many | 62,990 |
59 | wage | 62,931 |
60 | bank | 62,018 |
61 | rule | 61,256 |
… | … | … |
98 | real | 44,515 |
99 | china | 44,042 |
100 | every | 44,012 |
Rank | Word | TF-IDF Value |
---|---|---|
1 | blackfriday | 9.762917158451936 |
2 | craic | 9.762917158451936 |
3 | boo | 9.762917158451936 |
4 | bookings | 9.762917158451936 |
5 | acn | 9.762917158451936 |
6 | bookkeeper | 9.762917158451936 |
7 | adm | 9.762917158451936 |
8 | brave | 9.762917158451936 |
9 | aerospace | 9.762917158451936 |
10 | broadcom | 9.762917158451936 |
Rank | Word | Word Count |
---|---|---|
1 | emancipation | 99.52226201944997 |
2 | sabine | 99.44126423729803 |
3 | spikeproteins | 98.70374062769325 |
4 | willeford | 98.03656936906297 |
5 | tetrapod | 97.42748973013556 |
6 | mikayla | 97.42748973013556 |
7 | subsource | 96.34843497134476 |
8 | arbitration | 95.59369519372572 |
9 | delgado | 95.06230029904857 |
10 | advocateforrightsandknowledgeofamericansarkaghostio | 94.2598337269131 |
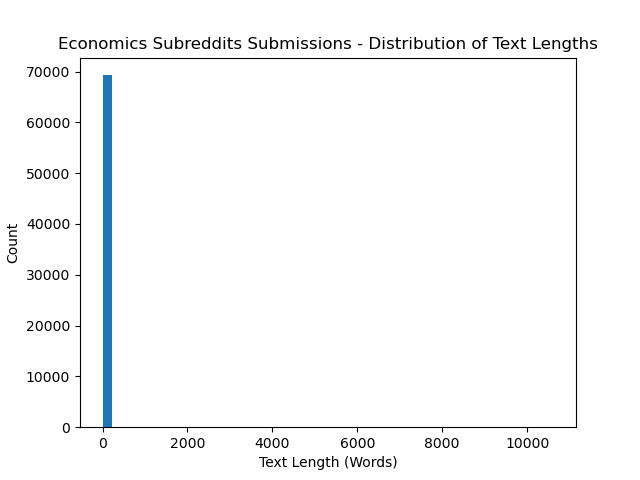
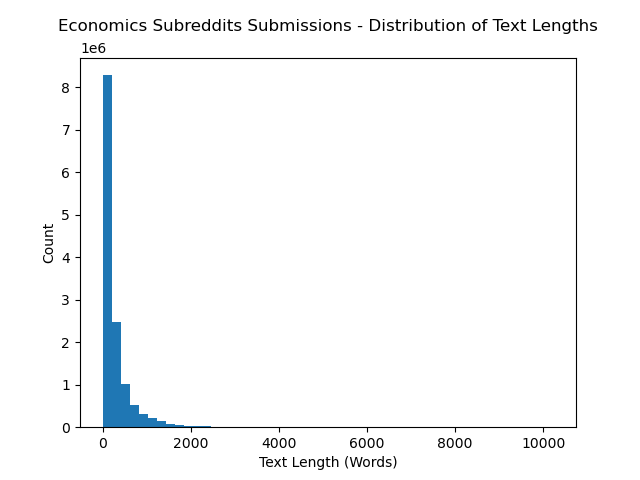
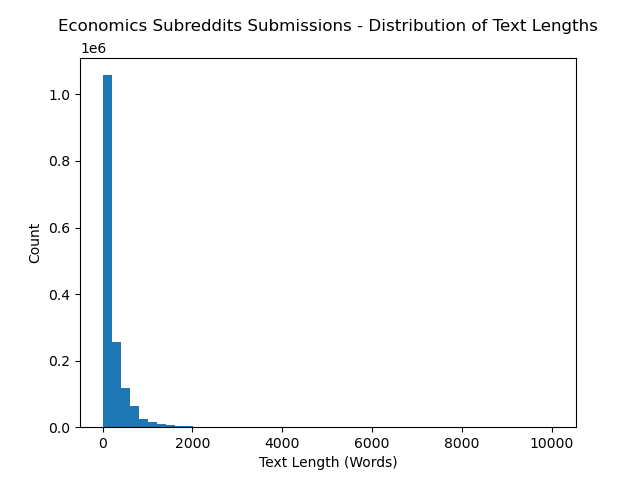